Reinforcement learning in Recommendation system
Reinforcement learning is how to make the actions to maximize the reward we will receive based on the situation (environment). It is exactly like the user react to recommendation system.
You can also check posts in this series:
In reinforcement environment, the learner must discover which way to achieve the highest reward by trying the actions without being told what to do. This action may affect not only the immediate reward but also the situation, then it will change the way to choose the action for our next choice.
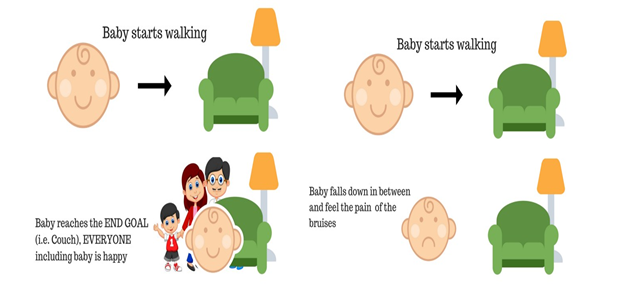
We can easily imagine that the reinforcement learning is similar to a baby trying to start walking. If he can walk step by step to his parents, he will receive compliments or gift, which is called positive reward. On the contrary, if he falls, he will feel pain and start to cry, which is called negative reward. However, because he was tempted by the parent’s gifts, the baby retries again and again till he gets the goal and continue to go farther. This is also the aim of a reinforcement learning system: trials-false till it can maximize its reward and continue to develop further. From the example of baby, we can model the reinforcement learning process like this:
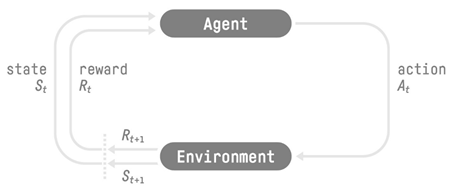
This process includes:
- Agent: like a baby, the agent will give out the action to the environment and then observe the state from the environment and receive the reward for its action.
- Environment: where the agent interacts with and return the reward and state.
- Action: like how the way baby choose to walk (short step or long step, step to right or step to left), this is agent’s decision to interact with the environment
- Reward: a result the agent receives from Environment, this result may be negative or positive.
- State: the agent will observe the state of it after interacting with the environment, just like a baby observe his position whether it is still far from his parents.
But other than agent and the environment, we can identify four main sub elements of RL.
- Policy: a core of agent that itself is sufficient to determine the behavior, mapping the states of the environment to actions to be taken when in those states.
- Reward: On each time step, the environment sends to the agent a single number called reward. The agent’s objective is to maximize the total reward it receives in long run. Thus, the reward signal defines what are the good and the bad signals for agent.
- Value Function: specifies the value of a state is the total amount of reward an agent can expect to accumulate over the future, starting from that state.
- Model of Environment: this mimics the behavior of the environment, that allows inferences to be made about how the environment will behave.
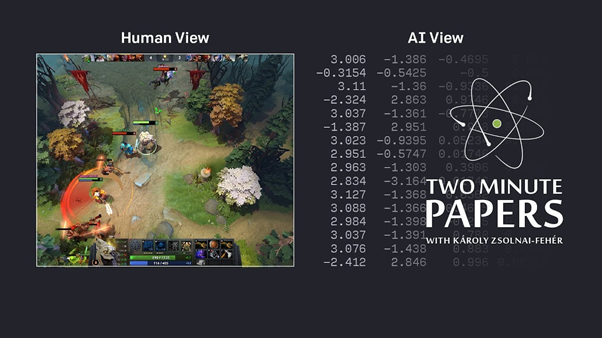
Reinforcement Taxonomies
Algorithms of reinforcement learning is divided into two groups: Model-Free and Model-Based.
- Model-based RL uses experience to construct an internal model of the transitions and immediate outcomes in the environment. Appropriate actions are then chosen by searching or planning in this world model.
- Model-free RL uses experience to learn directly one or both of two simpler quantities (state/ action values or policies) which can achieve the same optimal behavior but without estimation or use of a world model. Given a policy, a state has a value, defined in terms of the future utility that is expected to accrue starting from that state.
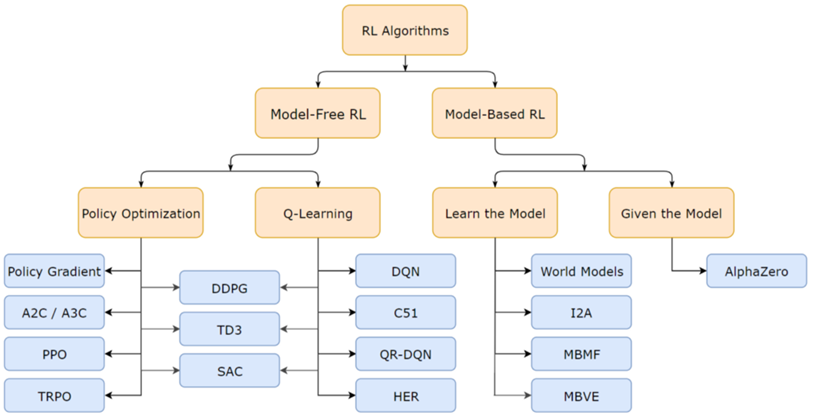
The picture above presents the summary of RL algorithms taxonomy. In the model-free side we have two type of algorithms are Policy Optimization (PO) and Q-Learning. Some algorithms of the first are Policy Gradient, Advanced-Actor-Critic (A2C), Asynchronous Advanced Actor-Critic (A3C), Proximal Policy Optimization (PPO) and Trust Region Policy Optimization (TRPO). With the latter, we have Deep Q-Learning (Q-Learning with Deep Learning) and some variants of DQN. However, we also have three algorithms which are the interference of PO and Q-Learning are Deep Deterministic Policy Gradient (DDPG), Soft Actor-Critic (SAC) and Twin Delay DDPG (TD3). About the Model-based RL, we now have some model from Deep Mind such as AlphaZero and some ideal model such as World Model (based on Variance Auto Encoder), Imagination-Augmented Agents (I2A, using LTSM with encoder)…, and more.
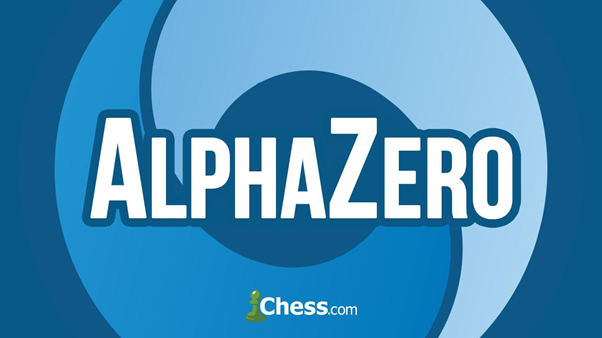
Useful resources:
Medium link: Reinforcement Learning Based Recommendation System – Part 2